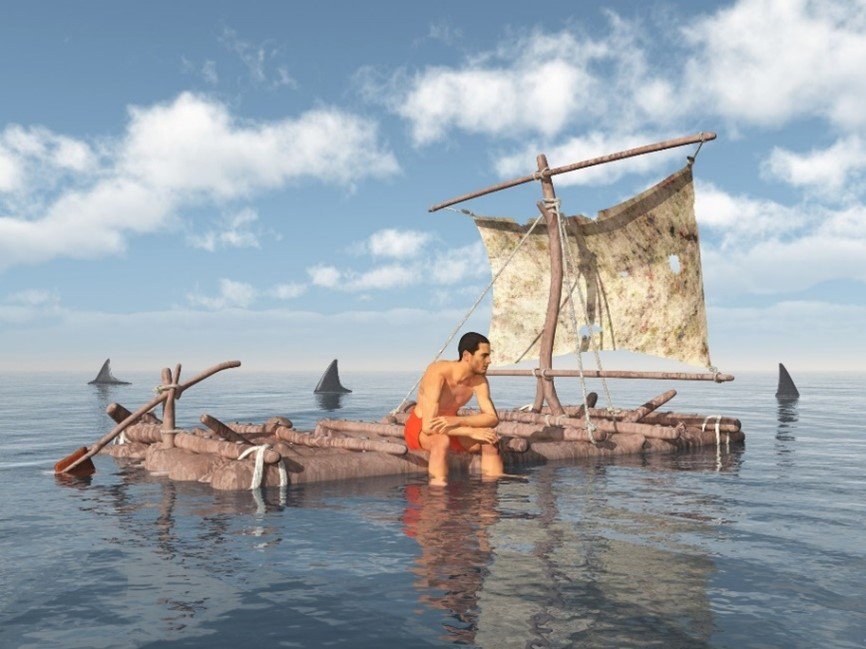
The often-misquoted line “Water, water everywhere, nor any drop to drink” from The Rime of the Ancient Mariner by Samuel Taylor Coleridge describes the irony of being surrounded by something yet not being able to derive any benefit from it. 225 years later, many companies find themselves in much the same situation: awash in vast amounts of data, but not experiencing the gains that had been promised by amassing all that information.
Where’s the disconnect? To answer that question we have to look back at how data storage came to exist in its present form.
Up until the first few years of the new millennium, data marts and larger data warehouses had become the standard for enterprise data storage. They were solid, dependable, readily accessible structures – but they were also rigid, relatively hard to scale, and becoming increasingly siloed. A strong point of data warehouses was their transform-then-load approach to aggregating data: schemas were created up front, and new data was first aligned with that pre-established schema and then loaded into the warehouse. It’s kind of like alphabetizing the spice jars in your kitchen: a lot of work up front, but then it’s very easy to find exactly what you’re looking for when you need it. However, with the volume and velocity of new data increasing all the time – requiring schemas to constantly be updated before new data could be loaded – this mechanism became unwieldy.
Around 2010 the data lake paradigm emerged. Data is poured into the lake in a load-then-transform approach. It’s quick – schemas don’t need to be updated every time a new data element appears – but the raw, unstructured storage doesn’t create much understanding about the data that has been gathered or the quality of any of that data. Back to the kitchen analogy, it’s quick and easy to come home from shopping and throw a bunch of new spice jars into a cabinet – but it will take more time to find what you’re looking for when you need it. And, because it’s hard to tell exactly what you have on hand, it’s very likely that you’re going to buy multiple jars of the same thing, which wastes money and takes up even more space.
Those two factors – money and space – were historically useful limiters and governors: storage space was expensive, so it had to be used wisely. That meant normalizing data so that multiple copies of the same element weren’t stored; it meant an efficient data schema so that related elements could quickly be retrieved and assembled. However, not long after the debut of the data lake, cloud storage became available – and it was cheap and plentiful. With data pouring in from an ever-increasing number of sources and now with virtually unlimited storage space available at a very low cost, the structure and efficiency of data marts and data warehouses gave way to the ‘just throw it in and we’ll worry about it later’ concept of data lakes.
Well, ‘later’ has a way of catching up to us – and now those same companies are trying to leverage all that accumulated data with the magic of AI and BI tools. And they’re finding that the magic isn’t quite powerful enough to find reliable features and novel insights in their data lake (which in many cases has eutrophicated into a data swamp.) It’s not the fault of the AI or BI tool – but in most cases those tools were developed in a laboratory or other highly refined setting, and trained on clean, orderly, relevant data. Unfortunately, the data that’s being fed to them in the real world isn’t clean and orderly (that wasn’t required in the data lake construct) and it’s far from relevant to a specific business problem or question, since the lake contains data from all over the enterprise.
Ironically, the giant, overfilled lake usually doesn’t contain unstructured data (e.g., sensor data, images, call recordings, .pdfs, chat logs, etc.) which might be especially relevant to the issue at hand – simply because that kind of non-transactional, non-financial data is often not included in what gets sent to the data lake. The ‘magic’ that companies are expecting from their AI or BI investment will frequently be found in those other data sources – and if the new tools can’t see the data, it’s no surprise that their performance isn’t as expected, and the investment is viewed as a disappointment.
The solution is straightforward: this is a case where more is not better. The data used to train an AI model or to be examined by a BI tool should be focused on a particular need / deliverable. The dataset won’t necessarily be compact (these tools are voracious) but it has to be relevant in order for the tools to work effectively and efficiently. There’s a tendency for clients to overlook their unstructured data at this point because they believe that data is inaccessible – and there’s an equal tendency for vendors not to bring up the subject if their clients don’t ask about it.
Before you embark on an AI or BI implementation – or if you’ve already done so and are disappointed with the outcome – keep the following in mind:
- Clearly define what you want this powerful new tool to do, and the business value that this investment will generate. If you can’t describe it in a sentence or two, with tangible results (e.g., an expected revenue gain, expense reduction, production efficiency improvement, etc.) you’re not ready. (And, no, “everyone else is using AI” doesn’t count as a business justification…)
- Determine exactly what data is needed to fulfill that goal. Be objective; be ruthless; include everything you need, and nothing more. Non-essential, ‘just-in-case’ data will only serve to slow down implementation, consume more resources, and not improve performance.
- Don’t forget to review your unstructured data (and this might be the first time your company is actually considering the use of that information). If your platform provider can’t label/annotate it properly, find a data services provider who can. If your platform still can’t ingest it, find another platform. There’s value trapped in your unstructured data; AI and BI tools can liberate it as long as the data is properly prepared.
We measure our most valuable commodities in small increments (ounces of gold, carats of diamonds). So it’s time to stop thinking about a company’s most valuable asset – its data – in broad terms of lakes and warehouses. Fill your AI or BI platform thoughtfully, drop by drop of precious data. You’ll find the result to be very refreshing.