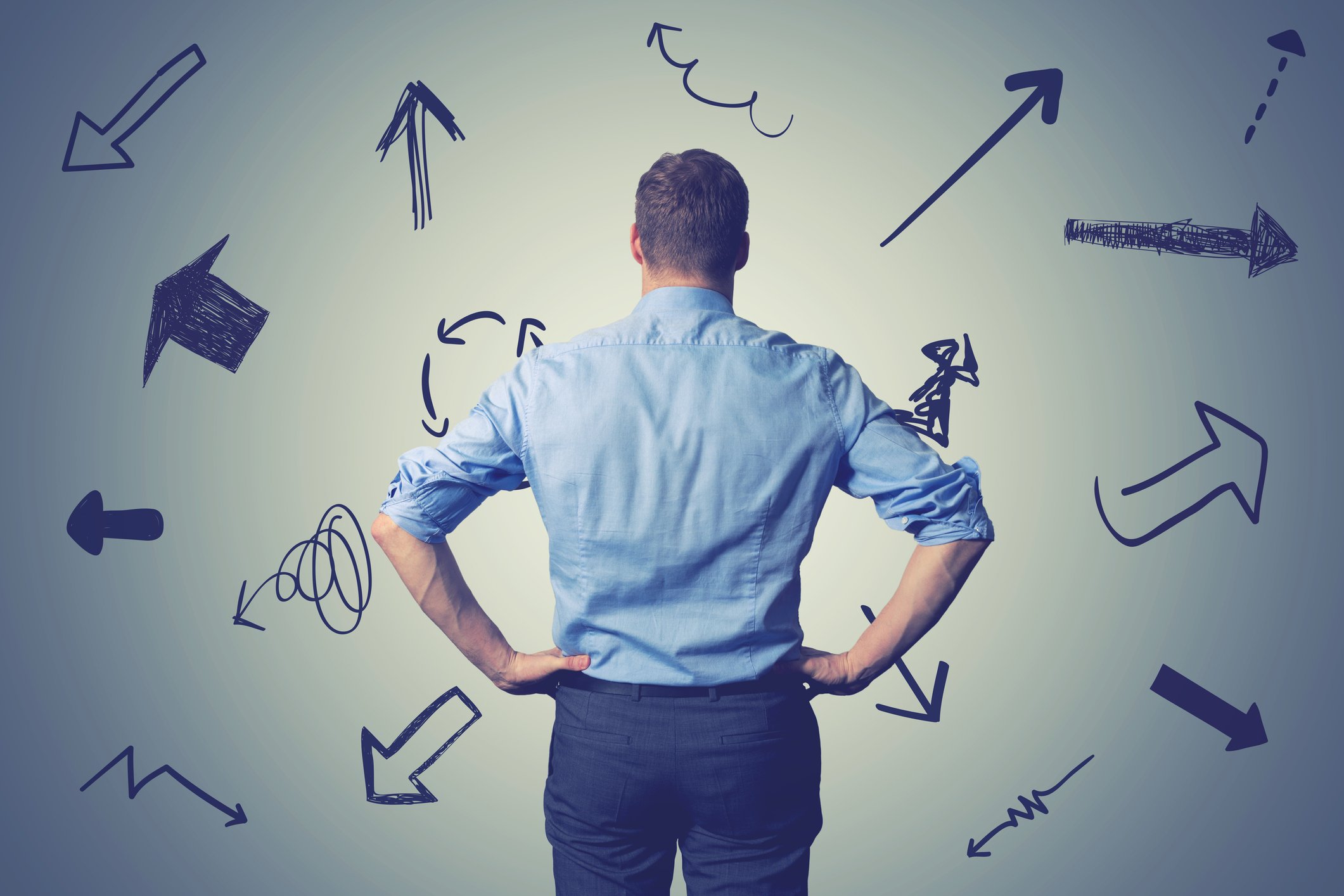
The news is filled with artificial intelligence and machine learning success stories showcasing groundbreaking results and new and innovative applications for the technology. What is less often reported, but just as notable, are the stories of disappointing returns on AI platform investments.
Organizations that are ready to jump into world of AI/ML should pause before making that leap.
According to the S&P 2023 Global Trends in AI Report, “More than two-thirds (69%) of surveyed organizations have at least one AI project in production (‘AI Pioneers’), whereas 31% of respondents’ AI projects are still in pilot or proof-of-concept stages (‘AI Explorers’). Additionally, 28% of survey respondents cite reaching enterprise scale with AI projects widely implemented and driving significant business value.” The study also notes, “Many organizations are acutely feeling the strain that AI models place on their data infrastructure. The increasingly large datasets required to train AI models are composed of diverse data types and have complex computation requirements, pushing the limits of traditional IT and data architectures.” (see the full report)
Those numbers highlight a significant disparity in the success rates of organizations that initiate an AI project and those that experience “significant business value” as a result. So why the gap in the success rate? It’s only after an AI/ML platform is purchased, that most organizations even begin to think about data including what data to apply to their business goals, how to gather high-quality data, how to interpret and label data, and how to analyze the results to ensure the correct data is being utilized. These are just some of the essential data considerations that many organizations fail to consider until their AI program fails to deliver results.
Organizations that want to join the ranks of the successful “AI pioneers” must take seriously the blend of ingredients that are essential to creating and maintaining an AI/ML initiative that yields significant business value. The key is to avoid the trap of creating an “AI strategy” and instead focus on using the power of AI to accelerate your existing business strategies.
Let’s look at each touchpoint along the path to a successful AI initiative:
Motivation. Why do you want an AI platform? Are you looking to boost profit margins, increase scale, or work more efficiently in a particular area? Organizations are purchasing and implementing AI to streamline operations, optimize processes and workflows, improve decision-making, personalize, and enhance the client experience, and ultimately gain a competitive edge. It’s critically important to understand your organization’s motivation to utilize AI so you can begin to formulate goals. A careful and honest analysis of your organization’s vision and mission for an AI/ML program should be discussed and made transparent by all decision-makers. It’s possible that after careful deliberation, an AI/ML program isn’t needed or may be premature to implement at this time.
Goals. What do you want to achieve from an AI or ML implementation? Are your organizational goals related to AI/ML aligned throughout the company? Unclear or undefined goals are one of the top reasons why an AI or ML program will fail. With all other AI program components running effectively, clear goals are necessary for the results to be accurately analyzed and salient conclusions reached. A lack of clearly defined business goals throughout your organization can undermine your ROI.
Technology. Artificial intelligence (AI) technology has been around since the 1950s. Since then, computer capability and machine learning (ML) algorithms have evolved to meet different applications and goals. New applications of AI and ML are being realized on what seems like a daily basis. System capability and accuracy are also increasing. Overall, the technology is sophisticated and largely accurate. However, human experts are essential to creating, maintaining, and improving all the fields associated with successfully deploying that foundational technology.
When it comes to your organization’s purchase and implementation of an AI platform, you’ll need to first make sure your internal technology systems can communicate and integrate with your new AI platform. Ensuring systems compatibility is essential to launching and maintaining a successful AI initiative that provides your organization with value, not headaches.
People. The next big decision is, who will do all the work required to generate optimal results from your technology investment? In other words, do you have the human resources to continually feed your AI program the data it needs to deliver on your identified goals? Artificial intelligence and machine learning cannot succeed without people. The truth is, behind every successful AI platform is a team of people making smart decisions about the raw data being fed into the platform. The only way machine learning systems can truly “learn” to accurately simulate human intelligence is by being fed complete and relevant data from the start. This is a continual process that can only be maintained by data curators–people specially trained to identify the high-value data that will be most useful to achieve progressively smarter and more accurate AI/ML. Does your organization have the human resources to do the work of organizing and analyzing your data? If you do not have an in-house data curation and analytics team, you need to strongly consider working with a third-party data specialist if you want to realize the full potential of your AI investment.
Data. Last, but certainly not least, is data–the fuel for all artificial intelligence platforms to function and machine learning to take place. When AI/ML programs fail to deliver the desired results, the culprit can sometimes be traced to incorrect data, incomplete data, biased data. But most likely, the failure is caused by underutilizing the organization’s unstructured data. Examples of unstructured data include: videos, images, audio, PDFs, email, social media posts, chat logs, scanned documents, and web insights.
Some questions to ask yourself as you implement an AI platform, are: Is my organization’s data easily available? Is my data mostly structured or unstructured? Am I prepared to master the production of clean data for input?
If your organization’s goal is to have your AI/ML program learn how to identify new trends in your business, you’ll need to identify all data sets having to do with trends and even data that may only appear tangentially related.
To reach this goal are you prepared to curate your unstructured and structured data for input into your AI/ML platform? This requires a team of people who are trained to meticulously label and annotate data and structure it so it can be fed into your models.
If you’re thinking that this sounds like a lot of work, it is. Acquiring or preparing labeled and annotated training data at scale is the single biggest challenge data science teams face. If your organization doesn’t master the data deliverables–large volumes of high-quality training data– your AI/ML program will fail.
Data is what trains all artificial intelligence models. Without data there is no machine learning. Humans working with data are the key to all successful AI platforms.
Unstructured Data is the Great Enabler of AI Success
If you’re only using structured data in your AI platform, your odds of success are significantly reduced. The lack of unstructured data has been identified as one of the main reasons many AI installations are not yielding the expected results.
It’s critical to keep in mind that AI and ML success relies on people and data. All forms of AI and ML, including generative AI and Predictive AI, rely on people to interpret and label an organization’s data points which are the fuel for learning.
Correctly formatting unstructured data into structured data takes precision and skill. That’s where DataInFormation℠ from Liberty Source comes in. The DataInFormation℠ suite of services helps clients by working with all types of unstructured data to identify the elements that will be most relevant to your company to help you meet your AI goals.
Studies show that even today’s most sophisticated AI and machine learning tools can only achieve a maximum of about 70% accuracy when processing images and unstructured text. Higher accuracy is possible with the right technology in the hands of experienced annotators to maximize the value of the data.
The DataInFormation℠ suite of solutions combines state-of-the-art labeling and annotation technology with human oversight and judgment to turn your unstructured data into structured data that’s ready to be integrated into your machine learning model. To accomplish this, a team of data engineers, process managers and skilled data associates use a combination of platforms and a great deal of experience to produce precision datasets that are essential to enable accurate machine learning.
If your machine learning platform isn’t performing as expected or you’re contemplating purchasing an AI or ML program, it’s time to consult a leading data labeling resource that can maximize the value of your organization’s data. DataInFormation℠ by Liberty Source provides data labeling, image annotation, natural language processing validation, computer vision calibration, training data curation and related data optimization services. These essential human-in-the-loop services are the key to clean, accurate data that can help prevent machine learning hallucinations from taking hold of your platform and ensure consistent performance, less downtime and re-work, and a higher return on your advanced technology investment.